
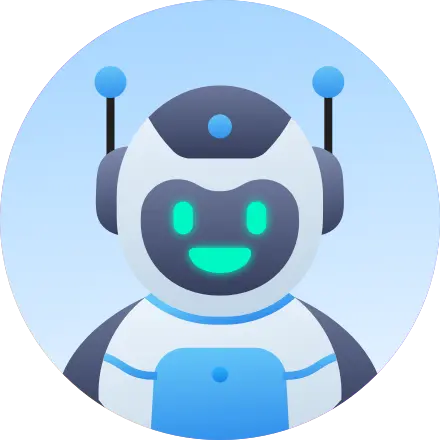



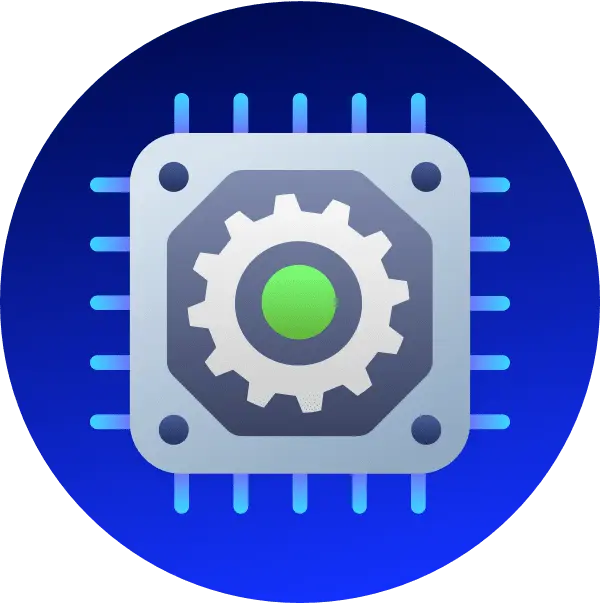


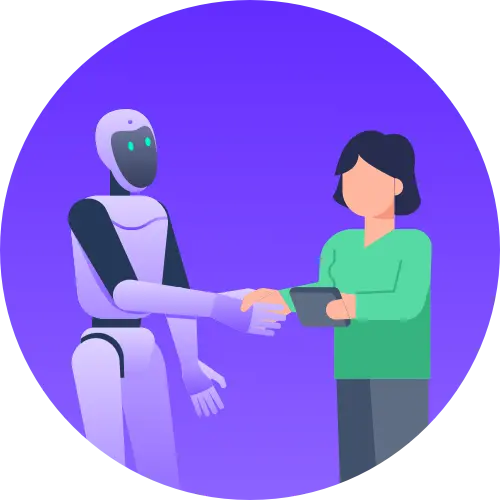
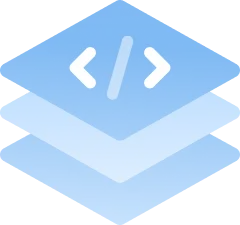
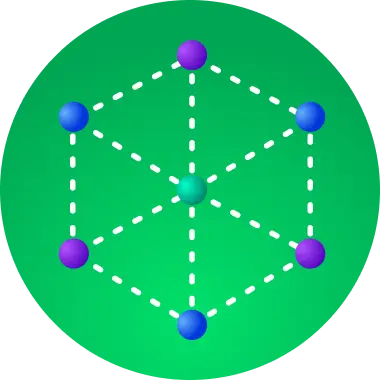
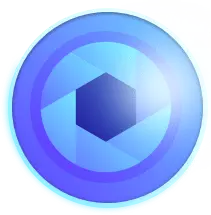
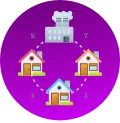

While organizations today might have large amounts of data, their datasets tend to be noisy, incomplete and imbalanced. This results in data scientists and engineers spending most of their precious time pre-processing, cleaning, and featurizing the data. These efforts are often insufficient, and deep learning techniques routinely fail on sparse datasets. Organizations are then forced to use classical machine learning techniques that require enormous amounts of manual feature engineering. At Abacus.AI, we are actively pursuing the following research areas that will enable training on less data.
Deep learning has seen great success across a wide variety of domains. The best neural architectures are often carefully constructed by seasoned deep learning experts in each domain. For example, years of experimentation have shown how to arrange bidirectional transformers to work well for language tasks and dilated separable convolutions for image tasks. A relatively new sub-field of deep-learning deals with automated machine learning, or as we prefer to call it: AI-assisted machine learning. The fundamental idea is that AI will create a first pass of the deep-learning model given a use-case or a dataset. Developers/data scientists can then either use that model directly or fine-tune. We are conducting cutting-edge research in the main pillars of AI-Assisted ML: hyperparameter optimization (HPO) and neural architecture search (NAS).
When developing a deep learning model, there are many knobs and dials to tune that
depend on the specific task and dataset at hand. For example, setting the learning rate
too high can prevent the algorithm from converging. Setting the learning rate too low
can cause the algorithm to get stuck at a local minimum. There are countless other
hyperparameters such as the number of epochs, batch size, momentum, regularization,
shape, and size of the neural network. These hyperparameters are all dependent on each
other and interact in intricate ways, so finding the best hyperparameters for a given
dataset is an extremely difficult and highly nonconvex optimization problem.
Randomly testing different sets of hyperparameters may eventually find a decent solution
but could take years of computation time. Efficiently tuning deep learning
hyperparameters is an active area of research. Five years ago, the best algorithms
weren’t much better than random search. Now algorithms are capable of orders of
magnitude speedups. At Abacus.AI, we use state-of-the-art HPO
while training all our models.
Neural architecture search (NAS) is a rapidly developing area of research in which the
process of choosing the best architecture is automated.
At Abacus.AI, we are using NAS to both fine-tune proven deep
network paradigms, and learn novel architectures for new domains. Our goal is to empower
data scientists and developers to create custom, production-grade models in days, not
months. See this blog post to read about our method, BANANAS,
which combines Bayesian optimization with neural predictors to
achieve state-of-the-art performance. Since making our code open-source, dozens of
developers have forked our repository, and two
independent research groups have confirmed that it achieves
state-of-the-art performance on NAS-Bench-101. BANANAS has even been cited in survey
papers on NAS.
We are also actively conducting fundamental research on the theory of NAS. Recently, we
studied local search for NAS - a simple yet effective
approach. We showed experimentally that local search gives state-of-the-art performance
on smaller benchmark NAS search spaces, but performs worse than random search on
extremely large search spaces. Motivated by this stark contrast, we gave a complete
theoretical characterization of local search. Our theoretical results confirm that local
search performs well on smaller search spaces and when the search space exhibits
locality.
Finally, we are conducting formal studies on the building blocks of NAS, including the
architecture
encoding. In most NAS algorithms, the neural architectures must be passed as
input to the algorithm using some encoding. For example, we might encode the neural
architectures using an adjacency matrix. Our recent work shows that this encoding can
have a substantial impact on the final result of the NAS algorithm. We conduct a set of
experiments with eight different encodings with various NAS algorithms. Our results lay
out recommendations for the best encodings to use in different settings within NAS.
Bias is one of the most important issues in machine learning today. Deep learning models are
being deployed in high-stakes scenarios today more than ever, and most of these models are found
to exhibit prejudices. For example, the New York Times reported that the majority of facial
recognition apps used by law enforcement agencies exhibit bias. They cited a study concluding
that facial recognition technology is ten times more likely to falsely identify people of color,
women and older people.
There has been considerable research in mitigating these biases, with dozens of definitions of bias and algorithms to decrease the level of
bias. The majority of fair algorithms are in-processing algorithms, which take as input a
training dataset and then train a new, fairer model from scratch. However, this is not always
practical. For example, recent neural networks such as XLNet or GPT-3 can take
weeks to train and are very expensive. Additionally, for some applications,
the full training set may no longer be available due to regulatory or privacy requirements.
At Abacus.AI, we are designing new post-hoc methods, which take as input a
pretrained model and a smaller validation dataset, and then debias the model through fine-tuning
or post-processing. We have designed three new techniques which work for applications with
tabular data or structured data. See our blog post for more information.
In addition to bias, we are actively working on explainability in neural networks. Business
Analysts and subject matter experts within organizations are often frustrated when dealing with
deep learning models. These models can appear to be black boxes that generate predictions which
humans can’t explain. Over the last two years, there has been considerable research in
explainability in AI. This has resulted in the release of an open-source tool, LIME,
which measures the responsiveness of a model’s outputs to perturbations in its inputs. Then
there’s SHAP (SHapley Additive exPlanations), a game-theoretic
approach to explain the output of any machine learning model. Google has introduced Testing with
Concept Activation Vectors (TCAV), a technique that may be used to generate insights and
hypotheses. Google Brain’s scientists also explored attribution of predictions to input features
in their 2016 paper, Axiomatic
attribution for deep neural networks. Our efforts in this area build on these techniques to
create a cloud microservice that will explain model predictions and determine if models exhibit
bias.